library(RColorBrewer)
display.brewer.all()
15 Colors
When evaluating the power and efficiency of a plot, color is always a key factor that sometimes speaks a language even louder than words. So in this chapter, you will be introduced with several widely-applied color schemes and get to know how to use proper colors to make better plots based on different features of your data.
15.1 RColorBrewer
RColorBrewer
is an R package having built-in sensible color schemes ready-to-use for figures. Colors are grouped into three types: sequential, diverging, and qualitative.
Sequential – Light colours for low data, dark for high data
Qualitative(for categorical data) – Colours designed to give maximum visual difference between categories so great for non-ordered categorical data
Diverging – Light colours for mid-range data, low and high use dark colours, great to seperate two extremes
Here is an example of plotting categorical data using Dark2
pallets under qualitative group of RColorBrewer:
library(ggplot2)
ggplot(iris, aes(Petal.Length, Sepal.Length, colour = Species)) +
geom_point() +
scale_colour_brewer(palette = "Dark2")
Also, you can create your own sequential pallets.
ggplot(faithfuld, aes(waiting, eruptions, fill = density)) +
geom_raster() +
scale_fill_gradient(low = "white", high = "red")
Or diverging pallets:
ggplot(faithfuld, aes(waiting, eruptions, fill = density)) +
geom_raster() +
scale_fill_gradient2(low = "grey", mid = "white", high = "red",midpoint = .02)
For discrete data, using scale_colour_manual
is a good choice. For discrete ordinal data, we can use another package (such as vcd)
ggplot(mtcars, aes(mpg, wt)) +
geom_point(aes(colour = factor(cyl))) +
scale_colour_manual(values = c("red", "yellow", "blue"))
<-brewer.pal(5,'Blues')
colorsbarplot(1:5, col=colors)
15.2 Perceptually uniform color spaces: Viridis
The viridis
R package provides four palettes for use in R which are pretty, perceptually uniform and easy to read by those with colorblindness.
The package contains eight color scales: viridis
, the primary choice, and five alternatives with similar properties - magma
, plasma
, inferno
, civids
, mako
, and rocket
-, and a rainbow color map - turbo
.
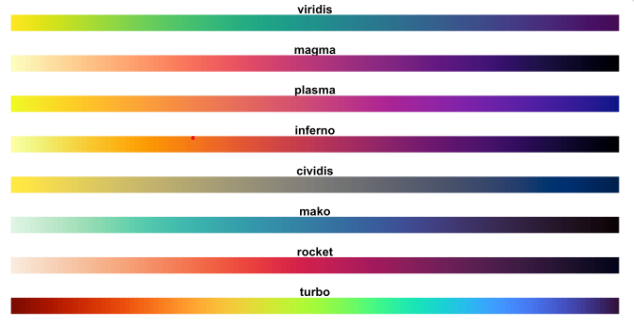
Perceived differences are proportional to scalar differences when using viridis
. The following example shows viridis
on continuous data using scale_color_viridis_c
, use scale_color_viridis_d()
for discrete data
library("viridis")
ggplot(iris, aes(Sepal.Length, Sepal.Width))+
geom_point(aes(color = Sepal.Length)) +
scale_color_viridis_c()